Talk of ‘Artificial Intelligence’ permeates the business landscape, but how viable is it in our industry?
By Thomas Paterson
Artificial intelligence and its applications are discussed everywhere—from refrigerators to drones in Ukraine and allegedly sentient conversation-bots. The IES 2022 Research Symposium addressed the question of where AI belongs in the lighting world. The following article is based on the opening keynote, looking at AI from the high level. Other speakers, published elsewhere, tackled individual applications in greater detail.
A working definition of intelligence, used by philosophers and scientists, is that “Humans are intelligent to the extent that our actions can be expected to achieve our objectives.” In machine intelligence, currently we’re only using tiny modules doing routine tasks. We’ve seen AI resolve simple objectives in daily life—“identify this face from a data pool,” “fill my washing machine moderately full and enough to wash these clothes.” Given the relative simplicity of the task, the agents are intelligent to the extent that they fulfill their tasks.
If our industry is going to use AI, we have to define clearly what its tasks would be, i.e., what are its objectives? Where would you want AI in lighting? Can you succinctly describe what success would involve?
Current applications of AI in lighting and likely in the near future include:
- Machine intelligence in design – input a building model, output a lighting design. For example, we can ask machines to lay out lighting in an office, to organize circuiting, to optimize systems at the design stage. For this, we need to set the project’s goals and let it work. It could produce the designs that we would otherwise do manually. This can relatively easily solve for IES light levels, architectural alignments and the like.
- Optimization of tasks. This is already happening in the built environment, for example in structural engineering to minimize steel usage. This uses basic, well understood algorithms with decades of application already.
- Construction can utilize AI for process and quality monitoring, doing comparisons between the built environment and the original design, as well as be part of robotics and other construction hardware.
- Operational systems can apply something simulating judgment in the operation of building systems, balancing lighting needs perhaps with views through shading, HVAC through temperature sensing and so on.
The majority of these applications resolve systematic, sequential processes and repetitive or data-intensive problems. Making kits of such solutions could be characterized as selling AI black boxes—installable modules that can inform how a lighting system operates, without the purchaser needing to know how the functions within the box actually operate. AI agents will struggle to deliver black boxes that serve complex functions such as applying human values intelligently; they will be best sold as simple energy management tools and the like. To achieve AI at higher levels of lighting application demands some cynicism. That is, with actions that can be expected to achieve our complex objectives, such as beauty, social activation, inclusion, etc.
These operational systems are where we’re seeing a lot of progress with energy optimization already a regular application, though the artificial intelligence paradigms are often barely more than branding. Applying 1990s neurofuzzy to establish the right light level during clouded moments is barely smarter than a washing machine filling to the approximately-right level.
When it comes to apparently sophisticated application, over recent months, we’ve seen the excitement around an intelligent agent, DALL-E (now called CrAIyon), producing images based on word descriptions. But it’s the weirdness and lack of applicability that makes the images compelling— and these were based on a training set of over 650,000 images and their captions. In lighting design we’re not documenting anything like this scale of training set; lighting designers document perhaps a few tens of thousands of designs a year. And even in something as simple as images in DALL-E (for which there are no coordination/integration/regulation requirements), we see endless weirdness.
Operationally, most hard data that we deal with in lighting is simple. A few hundred or a few thousand light levels, a few hundred data points—some sensors, timeclocks and solar models. The data sets are simple. Sensors and lights on the market with integrated AI agents are simple, and luminaire-level AI has probably largely run its course, essentially just relating ambient light or occupancy to dimming settings. We call this the “Illumination Devices Internet of Things” (IDIoT). This is essentially marketing hype without much merit to the AI usage over manually programmed algorithms.
“Smart is not something you install” is a classic phrase that encapsulates the idea that you have to design for a purpose and cannot simply buy something without context. Some AI can be embedded in simple systems, doing predictable tasks, but in small environments, the cost of AI specification and implementation means that payback periods are unachievable for tailored systems. Just as many small control systems end up being over-ridden and dumbed down, so too will custom AI be problematic. There is no scenario in which the energy savings generated by better light management will ever pay for the skills and time it takes to implement tailored intelligent systems in small offices.
At larger scale, this changes; the operators of full-size office towers and the like may find the payback comes close to being worthwhile. Especially once a system can spot those factors that are too subtle for a designer either to see or program, such as the predictable shadow sweep across a façade of windows from an adjacent building, balancing out peaks and troughs in natural light. The question will be: how big must a project become for tailored operational AI to justify its complexity?
To go beyond numerical tasks such as energy saving, visual comfort, circadian optimization, etc., we will need to define what we regard as the objectives of a lighting system. How does one code for aesthetics? Novelty of solutions? Social and business activation? Productivity? These are diverse and very human criteria. And to teach a system these objectives, we would have to code and quantify the outcomes of past systems that the new system can learn from.
As we look at the breadth of function of AI (as opposed to the sophistication of individual tasks), current applications serve very narrow intelligences. These are systems that are trained on data sets or optimization parameters that are provided by humans, and carry the same biases and outcome spaces as the models the humans inserted. It means that in, for example, the design space, a training set of past successful lighting schemes might simply train an agent to lay out efficient and appropriately spaced grids of downlights, ignoring the aesthetic and novelty criteria of professional design.
For practitioners, there are other concerns. Once we’ve designed or operated lighting systems via intelligence for a few years, will we end up so deskilled we can’t understand what they’re doing? We already see many young designers unable to do a lumen-method calculation or point calculation to validate if photometric software is performing. If an AI created an innovative lighting design that solved many criteria to an optimum, novel, solution, how would a design team recognize it? Could we tell the difference between something strange and peculiar but effective, and an error? The paradigm shift in the late 1990s from direct troffers to direct/indirect pendants as a typical typology for offices is an example of a step that would be hard to understand if an AI simply served it up without explanation. Acceptance depended on an increasing understanding of solutions to glare, visual comfort and healthy environments. If an AI is unable to explain a paradigm shift, it might look simply like an error, rather than a positive innovation. This is a joint challenge of both deskilling, and the inability to understand the underlying logic. This is the black-box problem—if you’re unable to understand what the AI is doing, how do you evaluate it?
Politically, and ethically, we will also have to consider privacy. AI for lighting application is not so much the threat here. The threat is that the data collection that is necessary for useful AI generates data sets that can be siphoned off for non-relevant and invasive usage.
The most likely high value for AI solutions may be in non-invasive optimization functions. For this, you look at data that is useful to client missions, available and correlative. At this stage there are few if any real-life AI applications of this sort of solution optimization, and we look to manual solutions to model how they could work. One example of useful correlative data is revenue, for example in the retail or hospitality sectors (see sidebar).
The test for the value of AI in this context would be what it takes to make the black box of machine intelligence smarter than a direct programming and able to generate faster/better returns on the investment. A study by McKinsey on the digitalization of the world, published early in 2022, projected that the next step in global digitalization and AI implementation will be a moment of “boom” in standardization—all building systems becoming interoperable. This could be considered the (sometimes wireless) universalization of blue wires. We already see this coming with Thread and Matter, platform standardization for IoT devices at the retail level, amid resistance from the incumbent players at the commercial scale.
As an industry, we need to look at what collective action we can take to empower valuable AI implementations that serve the industry and our clientele. The first place that lighting needs to move forward is in data aggregation and training sets. Huge aggregation of demonstrably successful systems will require both design documentation and related operational outcomes to be recorded. From there we can move on to operational systems that are able to understand how an environment is, or will perform, and optimize that performance. It may be tools that better help lay out lights; it may be operational systems that lower shades and turn up the interior lighting to reduce the amount of squinting people are doing. We will need built systems that log their inputs and outputs, generating the next generation of training sets. The more sophisticated the outputs tracked, the smarter the correlation and learning can be.
The data that these systems produce, at first, will be invaluable in developing subsequent generations of systems, which in turn will be collecting better data for subsequent generations. For now, we simply don’t have training sets for sophisticated functions because we’re not capturing correlated input and output data along with human valuation of the performance. We are just getting started on a very long journey.
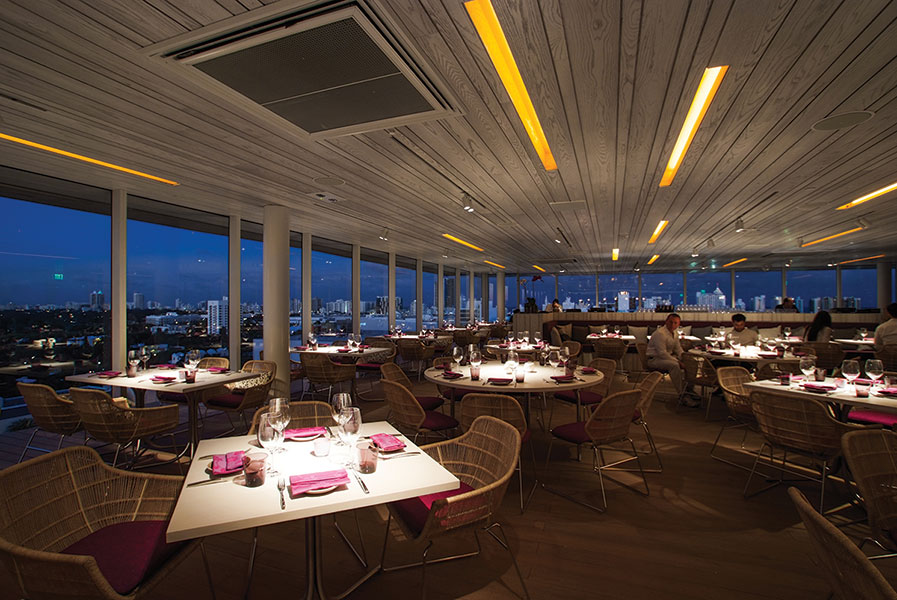
Cheers: AI Pays For Another Round
On a recent restaurant project Lux Populi wanted to optimize revenue and its correlation with light levels. Designers programmed distinct approaches with different contrast levels, general versus table illumination, and particularly, transitions at different times of the evening. Designers then did A/B/C testing over three months and were able to deliver a delta of $1,800 per night between the best and worst scene settings. The test identified the implicit tastes of the demographic who were eating there, and as a result, the design plan generated an extra cocktail sale per table, on average. This was done manually, but an intelligent agent could explore to find the same results. It would need to work out what parameters were relevant and impactful, which would probably require a human to tell the system “where to look.”